SURF/SIFT特征描述
视频讲解如下:
在本章节中给大家演示SURF/SIFT特征描述,主要函数:SURF和SIFT。
毛星云的代码为opencv-2.4.9版本的SURF功能演示,可是博主在opencv-2.4.9版本中并未发现SURF功能,目前只能演示SIFT了。
C#、C++版本中提供了Detect和DetectAndCompute两种算法,效果其实也都差不多,博主代码中两种操作方法都提供了,Python版本中好像好像无法使用Detect,执行后返回结果是空的。。。
当前系列所有demo下载地址:
https://github.com/GaoRenBao/OpenCv4-Demo
不同编程语言对应的OpenCv版本以及开发环境信息如下:
语言 | OpenCv版本 | IDE |
C# | OpenCvSharp4.4.8.0.20230708 | Visual Studio 2022 |
C++ | OpenCv-4.5.5-vc14_vc15 | Visual Studio 2022 |
Python | OpenCv-Python (4.6.0.66) | PyCharm Community Edition 2022.1.3 |
首先呢,我们需要准备两张测试图片。(不想下载工程的童鞋,可直接复制下面的图片和代码)
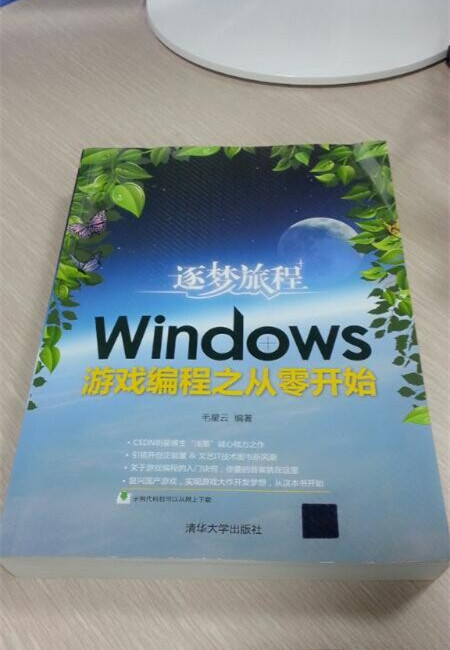
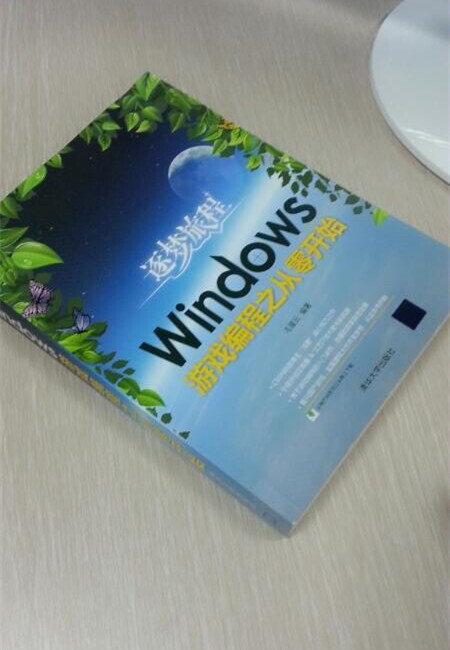
C++ SIFT版本运行效果如下(其他版本效果也差不多):
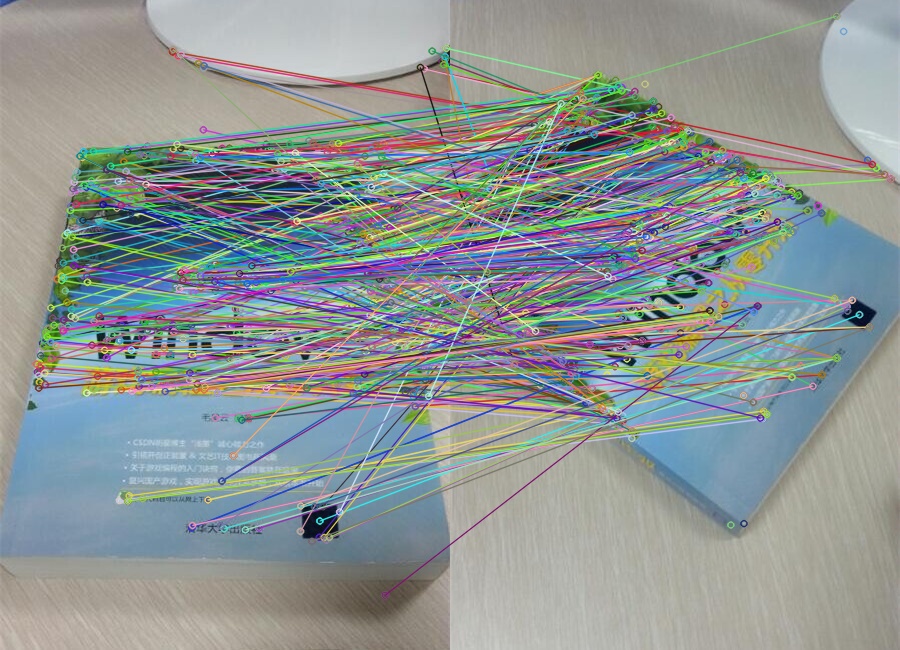
C#版本代码如下:
using OpenCvSharp;
namespace demo
{
internal class Program
{
static void Main(string[] args)
{
demo1();
demo2();
Cv2.WaitKey();
}
/// <summary>
/// SURF
/// </summary>
static void demo1()
{
// 载入源图片并显示
Mat srcImage1 = Cv2.ImRead("../../../images/book2.jpg");
Mat srcImage2 = Cv2.ImRead("../../../images/book3.jpg");
// 定义SURF中的hessian阈值特征点检测算子
int minHessian = 700;
// 定义一个特征检测类对象
var MySurf = OpenCvSharp.XFeatures2D.SURF.Create(minHessian, 4, 3, true, true);
Mat descriptors1 = new Mat();
Mat descriptors2 = new Mat();
// 模板类是能够存放任意类型的动态数组,能够增加和压缩数据
// 方法1:计算描述符(特征向量),将Detect和Compute操作分开
//KeyPoint[] keyPoint1 = MySurf.Detect(srcImage1);
//KeyPoint[] keyPoint2 = MySurf.Detect(srcImage2);
//MySurf.Compute(srcImage1, ref keyPoint1, descriptors1);
//MySurf.Compute(srcImage2, ref keyPoint2, descriptors2);
// 方法2:计算描述符(特征向量),将Detect和Compute操作合并
KeyPoint[] keyPoint1, keyPoint2;
MySurf.DetectAndCompute(srcImage1, null, out keyPoint1, descriptors1);
MySurf.DetectAndCompute(srcImage2, null, out keyPoint2, descriptors2);
// 使用BruteForce进行匹配
// 创建特征点匹配器
BFMatcher matcher = new BFMatcher();
// 匹配两幅图中的描述子(descriptors)
DMatch[] matches = matcher.Match(descriptors1, descriptors2);
//【6】绘制从两个图像中匹配出的关键点
Mat imgMatches = new Mat();
Cv2.DrawMatches(srcImage1, keyPoint1, srcImage2, keyPoint2, matches, imgMatches);//进行绘制
// 显示效果图
Cv2.ImShow("SURF匹配图", imgMatches);
}
/// <summary>
/// SIFT
/// </summary>
static void demo2()
{
// 载入源图片并显示
Mat srcImage1 = Cv2.ImRead("../../../images/book2.jpg");
Mat srcImage2 = Cv2.ImRead("../../../images/book3.jpg");
// 定义SURF中的hessian阈值特征点检测算子
int minHessian = 700;
// 定义一个特征检测类对象
var MySift = OpenCvSharp.Features2D.SIFT.Create(minHessian);
Mat descriptors1 = new Mat();
Mat descriptors2 = new Mat();
// 模板类是能够存放任意类型的动态数组,能够增加和压缩数据
// 方法1:计算描述符(特征向量),将Detect和Compute操作分开
//KeyPoint[] keyPoint1 = MySift.Detect(srcImage1);
//KeyPoint[] keyPoint2 = MySift.Detect(srcImage2);
//MySift.Compute(srcImage1, ref keyPoint1, descriptors1);
//MySift.Compute(srcImage2, ref keyPoint2, descriptors2);
// 方法2:计算描述符(特征向量),将Detect和Compute操作合并
KeyPoint[] keyPoint1, keyPoint2;
MySift.DetectAndCompute(srcImage1, null, out keyPoint1, descriptors1);
MySift.DetectAndCompute(srcImage2, null, out keyPoint2, descriptors2);
// 使用BruteForce进行匹配
// 创建特征点匹配器
BFMatcher matcher = new BFMatcher();
// 匹配两幅图中的描述子(descriptors)
DMatch[] matches = matcher.Match(descriptors1, descriptors2);
//【6】绘制从两个图像中匹配出的关键点
Mat imgMatches = new Mat();
Cv2.DrawMatches(srcImage1, keyPoint1, srcImage2, keyPoint2, matches, imgMatches);//进行绘制
// 显示效果图
Cv2.ImShow("SIFT匹配图", imgMatches);
}
}
}
C++版本代码如下:
#include <iostream>
#include <opencv2/opencv.hpp>
#include <opencv2/core/core.hpp>
#include <opencv2/features2d.hpp>
#include <opencv2/highgui/highgui.hpp>
#include <opencv2/features2d/features2d.hpp>
using namespace cv;
using namespace std;
int main()
{
//【0】改变console字体颜色
system("color 1F");
//【1】载入素材图
Mat srcImage1 = imread("../images/book2.jpg");
Mat srcImage2 = imread("../images/book3.jpg");
if (!srcImage1.data || !srcImage2.data)
{
printf("读取图片错误,请确定目录下是否有imread函数指定的图片存在~! \n"); return false;
}
//【2】定义SIFT中的hessian阈值特征点检测算子
int minHessian = 700;
Ptr<SIFT> siftDetector = SIFT::create(minHessian);
//vector模板类,存放任意类型的动态数组
vector<KeyPoint> keyPoint1, keyPoint2;
Mat descriptors1, descriptors2;
//【4】方法1:计算描述符(特征向量),将Detect和Compute操作分开
siftDetector->detect(srcImage1, keyPoint1);
siftDetector->detect(srcImage2, keyPoint2);
siftDetector->compute(srcImage1, keyPoint1, descriptors1);
siftDetector->compute(srcImage2, keyPoint2, descriptors2);
//【4】方法2:计算描述符(特征向量),将Detect和Compute操作合并
//siftDetector->detectAndCompute(srcImage1, cv::Mat(), keyPoint1, descriptors1);
//siftDetector->detectAndCompute(srcImage2, cv::Mat(), keyPoint2, descriptors2);
//【5】使用BruteForce进行匹配
// 创建特征点匹配器
Ptr<BFMatcher> matcher = BFMatcher::create();
// 匹配两幅图中的描述子(descriptors)
vector<DMatch> matches;
matcher->match(descriptors1, descriptors2, matches);
//【6】绘制从两个图像中匹配出的关键点
Mat imgMatches;
drawMatches(srcImage1, keyPoint1, srcImage2, keyPoint2, matches, imgMatches);//进行绘制
//【7】显示效果图
imshow("匹配图", imgMatches);
waitKey(0);
return 0;
}
Python版本代码如下:
import cv2
import numpy as np
# 【1】载入图像
srcImage1 = cv2.imread("../images/book2.jpg")
srcImage2 = cv2.imread("../images/book3.jpg")
# 定义一个特征检测类对象
sift = cv2.SIFT_create(700)
# 调用detect函数检测出SIFT特征关键点,保存在vector容器中
# 方法1:计算描述符(特征向量),将Detect和Compute操作分开
keyPoint1 = sift.detect(srcImage1)
keyPoint2 = sift.detect(srcImage2)
(keypoints1, descriptors1) = sift.compute(srcImage1, keyPoint1)
(keypoints2, descriptors2) = sift.compute(srcImage2, keyPoint2)
# 方法2:计算描述符(特征向量),将Detect和Compute操作合并
#(keyPoint1, descriptors1) = sift.detectAndCompute(srcImage1, None)
#(keyPoint2, descriptors2) = sift.detectAndCompute(srcImage2, None)
matcher = cv2.BFMatcher() #建立匹配关系
matches=matcher.match(descriptors1,descriptors2) #匹配描述子
matches=sorted(matches,key=lambda x:x.distance) #据距离来排序
#画出匹配关系
outImg = None
imgMatches = cv2.drawMatches(srcImage1, keyPoint1,srcImage2,keyPoint2,matches, outImg = None)
#imgMatches = cv2.drawMatches(srcImage1, keyPoint1,srcImage2,keyPoint2,mathces[:40], outImg = None)
cv2.imshow("imgMatches", imgMatches)
cv2.waitKey(0)
cv2.destroyAllWindows()